The Best Image Labeling Tool: Revolutionizing Software Development
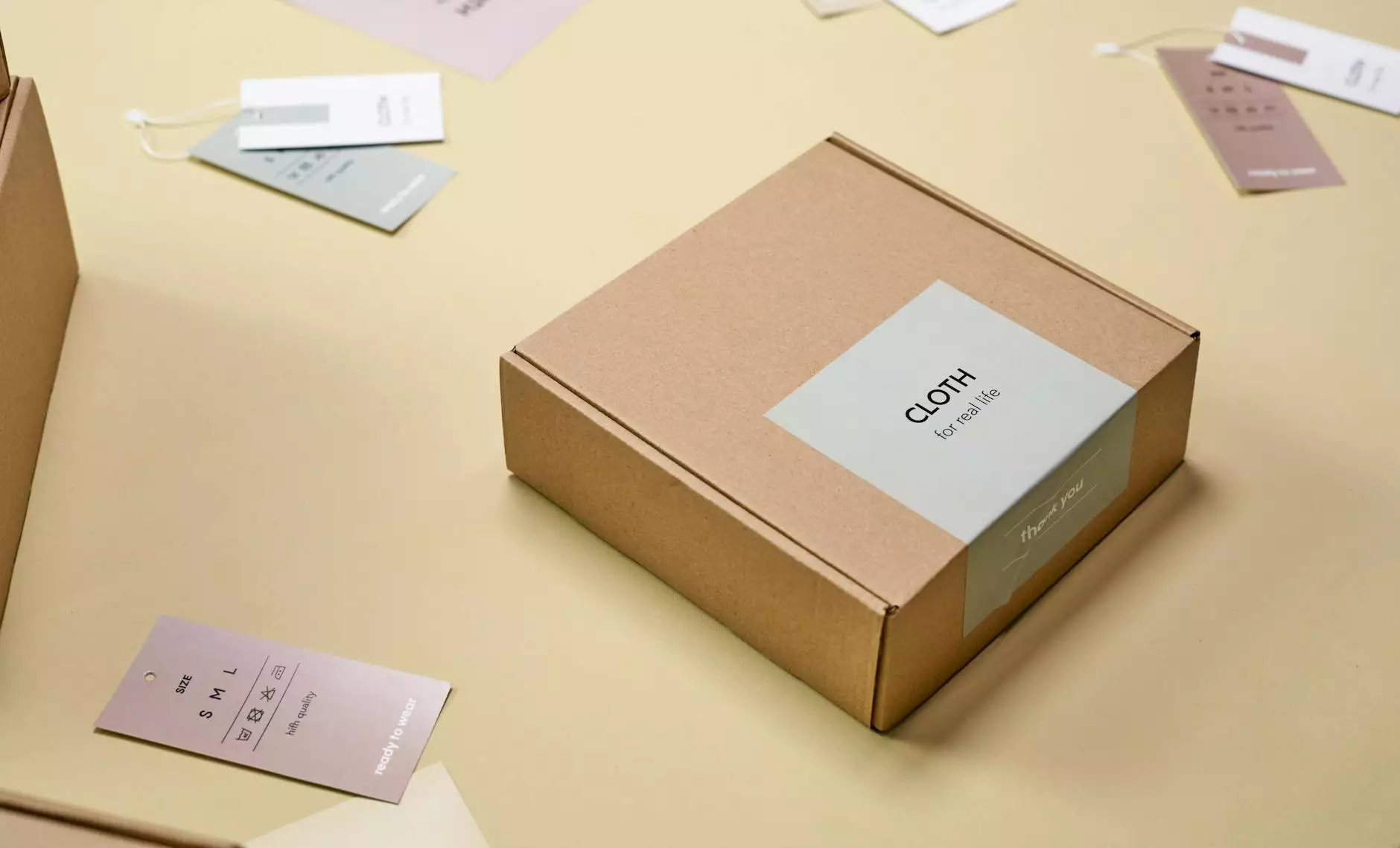
In today's fast-evolving digital landscape, effective image labeling has emerged as a vital element in software development, particularly in fields like artificial intelligence, machine learning, and computer vision. For developers and businesses looking to thrive in this domain, finding the best image labeling tool is essential. This comprehensive guide will explore the significance of image labeling, the features that define the top tools in the market, and how they can streamline your software development processes.
Understanding the Importance of Image Labeling
Image labeling refers to the process of annotating images to provide contextual information related to the objects, scenes, and elements within them. Properly labeled images serve as a foundational dataset for training machine learning models, enabling computers to recognize and interpret visual data. Here are a few reasons why image labeling is crucial:
- Data Accuracy: Proper image labeling improves the accuracy of machine learning models, leading to better performance in real-world applications.
- Enhanced Recognization: Labeled datasets allow models to learn features and patterns, which enhances their ability to recognize and classify new images.
- Improved Model Training: Well-labeled images contribute to the effectiveness of training algorithms, reducing the chances of errors and increasing reliability.
- Time and Cost Efficiency: Automating the labeling process with the right tools can save valuable time and resources in software development projects.
Characteristics of the Best Image Labeling Tools
The search for the best image labeling tool can be daunting given the myriad of options available. However, certain features can help guide your decision:
1. User-Friendly Interface
The best image labeling tools present an intuitive and user-friendly interface. This feature is essential for teams to quickly learn the tool and focus on efficient labeling without steep learning curves.
2. Versatility in Labeling Options
Investing in a labeling tool that offers various annotation types such as bounding boxes, polygons, points, and semantic segmentation options is crucial. This versatility accommodates a wide range of use cases, from simple object detection to complex scene parsing.
3. Automation Features
Incorporating automation features, such as AI-assisted labeling, dramatically speeds up the annotation process by suggesting labels or even handling entire images. This capability significantly reduces the time required for large-scale projects.
4. Collaboration Tools
Given that image labeling often involves teams, the best tools integrate collaboration features allowing multiple users to work simultaneously. Real-time updates and communication tools also enhance team efficiency.
5. Integration with Other Software
The best image labeling tool should integrate seamlessly with existing software development environments and machine learning frameworks. APIs and export options for commonly used file formats facilitate smooth workflows.
6. Secure and Scalable
As data privacy becomes increasingly critical, selecting a labeling tool that prioritizes security and offers scalability options is essential for businesses of all sizes. Look for tools that comply with industry standards and regulations.
Comparative Analysis of Top Image Labeling Tools
To help you make an informed choice, we have conducted a comparative analysis of some of the top image labeling tools available today:
A. Keymakr
Keymakr stands out as a leading image labeling tool renowned for its robust feature set tailored to improve labeling efficiency and accuracy. Keymakr’s intuitive interface and multiple labeling options, including bounding and polygon annotations, make it a preferred choice for businesses.
Key Features:
- User-friendly design
- AI-assisted labeling features
- Export in various formats, including COCO and Pascal VOC
- Collaboration tools for real-time work
- Flexible pricing plans suitable for any business scale
B. Labelbox
Labelbox provides a comprehensive platform that combines image labeling with project management tools. It’s particularly favored for its strong automation capabilities, allowing teams to label large datasets quickly.
Key Features:
- Collaboration features for teams
- Powerful automation options
- Software integrations with popular tools
- Analytics dashboards for performance tracking
C. Supervisely
Supervisely is a robust platform specifically designed for computer vision projects. It offers advanced functionalities for advanced users who require in-depth annotation options tailored for AI-driven initiatives.
Key Features:
- Rich annotation interfaces for various data types
- Support for multiple data formats and export options
- Powerful AI training capabilities
- Community-driven improvements and plugins
Choosing the Right Tool for Your Business
Determining the best image labeling tool for your business involves a few critical steps:
1. Assess Your Needs
Evaluate the specific requirements of your projects. Consider the types of images you will be labeling, the size of your datasets, and how often you will need to update annotations.
2. Budget Considerations
Establish a budget for your labeling tool. There are free options, but premium tools often provide additional features that can significantly improve your workflow.
3. Trial Periods
Take advantage of trial versions before making a commitment. This step will allow you to assess how well the tool integrates into your team’s workflow and whether it meets your expectations.
Implementing Best Practices for Effective Image Labeling
While choosing the right image labeling tool is vital, implementing best practices will enhance high-quality outputs:
1. Standardization of Labels
Create a standardized labeling guideline that clearly defines what each label represents. Consistency is key to maintaining accuracy throughout the dataset.
2. Continuous Training
Regular training sessions for your team on the selected tool and annotation guidelines can improve efficiency and reduce errors in the labeling process.
3. Regular Quality Checks
Implement routine quality checks to verify the accuracy of labeled images. Spot-checking annotations can prevent potential issues down the line in model training.
4. Feedback Loops
Establish feedback mechanisms to assess the labeling process continually. Learning from past projects can help improve future labeling efforts.
Conclusion
The hunt for the best image labeling tool concludes with a careful evaluation of available options, an understanding of your unique needs, and the implementation of effective labeling practices. Choosing a reliable tool such as Keymakr can drastically enhance your software development cycle by streamlining data preparation, improving model performance, and ultimately contributing to the success of your AI projects. Invest in the right tools today and transform your image labeling processes for better results tomorrow.